Invited Talks
The conference will take place from 25th to 28th November 2024. Below you find the schedule of the invited talks for each day. See also the Schedule overview, Main Track Schedule, Workshops Schedule, Tutorials, and Awards/Schools/Industry session pages.
Representation learning for acting and planning
Tuesday 26th November
9:00 – 10:00
Hector Geffner
RWTH Aachen University
Chair: Marco Montali
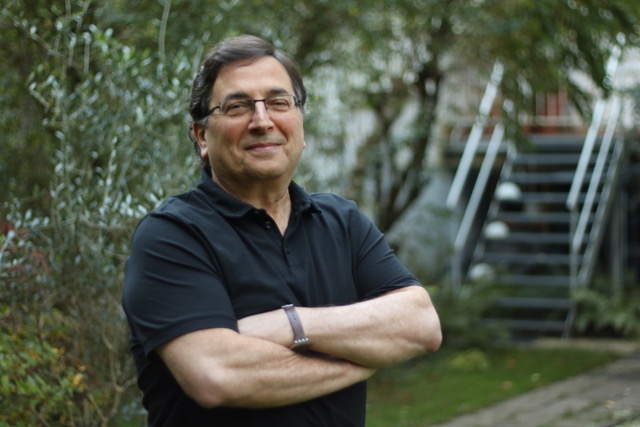
Abstract
Recent progress in deep learning and deep reinforcement learning (DRL) has been truly
remarkable yet two key problems remain: structural policy generalization and policy reuse.
The first is about getting policies that generalize in a reliable way; the second is about
getting policies that can be reused and combined in a flexible, goal-oriented manner. The
two problems are studied in DRL but only experimentally, and the results are not crisp and clear.
In our work, we have tackled these problems in a slightly different way, separating what is
to be learned from how. For this, we have developed languages for expressing, studying,
and learning general policies, using combinatorial and DRL approaches. We have also developed
languages and methods for expressing, studying and learning general subgoal structures and
hierarchical polices. Open challenges and general methodological lessons will be discussed.
This is joint work with Blai Bonet, Simon Stahlberg, Dominik Drexler, and other members of the RLeap team.
About the speaker
Hector Geffner is an Alexander von Humboldt Professor at the RWTH Aachen University, Germany.
Before joining RWTH in 2023, he was an ICREA Research Professor at the Universitat Pompeu Fabra in Barcelona, Spain. Hector obtained a Ph.D. in Computer Science at UCLA and worked at the IBM T.J. Watson Research Center in New York and at the Universidad Simon Bolivar in Caracas. Distinctions for his work include the 1990 ACM Dissertation Award and three ICAPS Influential Paper Awards. He currently leads a project on representation learning for acting and planning (RLeap) funded by an ERC grant.
The Language Factor
Wednesday 27th November
09:00 – 10:00
Malvina Nissim
University of Groningen
Chair: Alessandro Artale
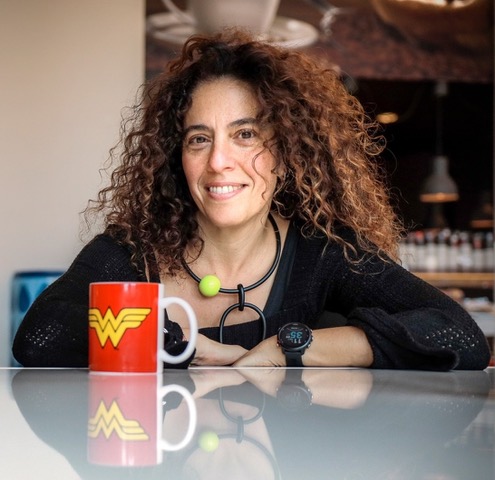
Abstract
Large Language Models, arguably more than any other development until now, have made Artificial Intelligence a general public affair. Although it appears that everybody has something to say about LLMs, possibly also because language truly is everyone’s ability and daily business, language itself is far from being the primary interest of those interested in LLMs. This talk will take a different take, and through modelling experiments, personal reflections, and interactions with the audience we’ll put language on the throne it deserves in the realm of LLMs (and evaluate its role in current developments.)
About the speaker
Malvina Nissim (https://malvinanissim.github.io) is full professor of Computational Linguistics & Society at the University of Groningen, The Netherlands, where she is a member of the GroNLP (Groningen Natural Language Processing) group. Before settling in Groningen over 10 years ago, she lived, studied, and worked in Italy (where she grew up), Scotland, and Germany. She works on multiple aspects of Natural Language Processing, with particular attention to language itself and the interaction of Language Technology and society. Relatedly, she is the coordinator of the Sectorplan‘s theme Humane AI at the University of Groningen, and is a member of the ACL Ethics Committee. As a complement to her research activities she is keen to engage in science communication and outreach, including to school children, especially towards an aware and responsible use of language technologies. She very much enjoys public speaking, and she was lucky to get the chance to give a TEDx talk and to be invited by the European Council to give a plenary lecture on gender bias in Artificial Intelligence on International Women’s Day 2021. As a university professor she teaches quite a bit and was elected as the 2016 University of Groningen Lecturer of the Year. Malvina is also a member of the Scientific Advisory Board of the Jantina Tammes School for Digital Society, Technology, and AI at the University of Groningen, and a member of the board of AILC, the Italian Association for Computational Linguistics. To survive a full time job she loves to run near the water, to knit, and to spend time with her two teenagers.
Against the clock: lessons learned by applying temporal planning in practice
Thursday 28th November
09:00 – 10:00
Andrea Micheli
Fondazione Bruno Kessler, Trento
Chair: Gabriella Cortellessa
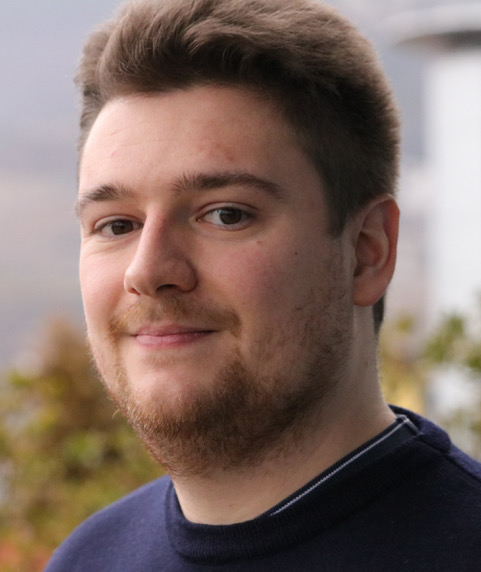
Abstract
Automated Planning is a foundational area of AI research, focusing on the automated synthesis of courses of actions to achieve a desired goal within a formally-modeled system. When dealing with time and temporal constraints, this problem is known as Temporal Planning. In this talk, I will present my research on the application of temporal planning to real-world scenarios, and highlight the open research directions in this field. Starting from a series of projects in different application domains —including robotics, manufacturing, and logistics—I will explore key challenges encountered, the (sometimes hard) lessons learned, and the techniques, tools, and methodologies that have emerged from these efforts. Additionally, I will introduce and discuss preliminary results on applying Reinforcement Learning techniques to tailor temporal planners to specific application contexts.
About the speaker
Andrea Micheli (https://andrea.micheli.website) is the head of the “Planning Scheduling and Optimization” research unit at Fondazione Bruno Kessler, Trento, Italy (https://pso.fbk.eu). His research focuses on the development and technology transfer of automated planning technologies. He obtained his PhD in Computer Science from the University of Trento in 2016. His PhD thesis titled “Planning and Scheduling in Temporally Uncertain Domains” won several awards including the EurAI Best Dissertation Award and the honorable mention at the ICAPS Best Dissertation award. He currently works in the field of temporal planning and is the main developer of the TAMER planner (tamer.fbk.eu). He is also lead developer of the pysmt (https://www.pysmt.org) open-source project aiming at providing a standard Python API for satisfiability modulo theory solvers. Andrea coordinated the AIPlan4EU project (https://www.aiplan4eu-project.eu) aiming to remove the access barriers to automated planning technology and to bring such technology to the European AI On-Demand Platform. He authored more than 30 papers in the Formal Methods and Artificial Intelligence fields. Andrea recently won an ERC Starting Grant (https://pso.fbk.eu/articles/step-rl) for researching novel solutions in the combination of temporal planning and reinforcement learning.